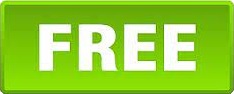
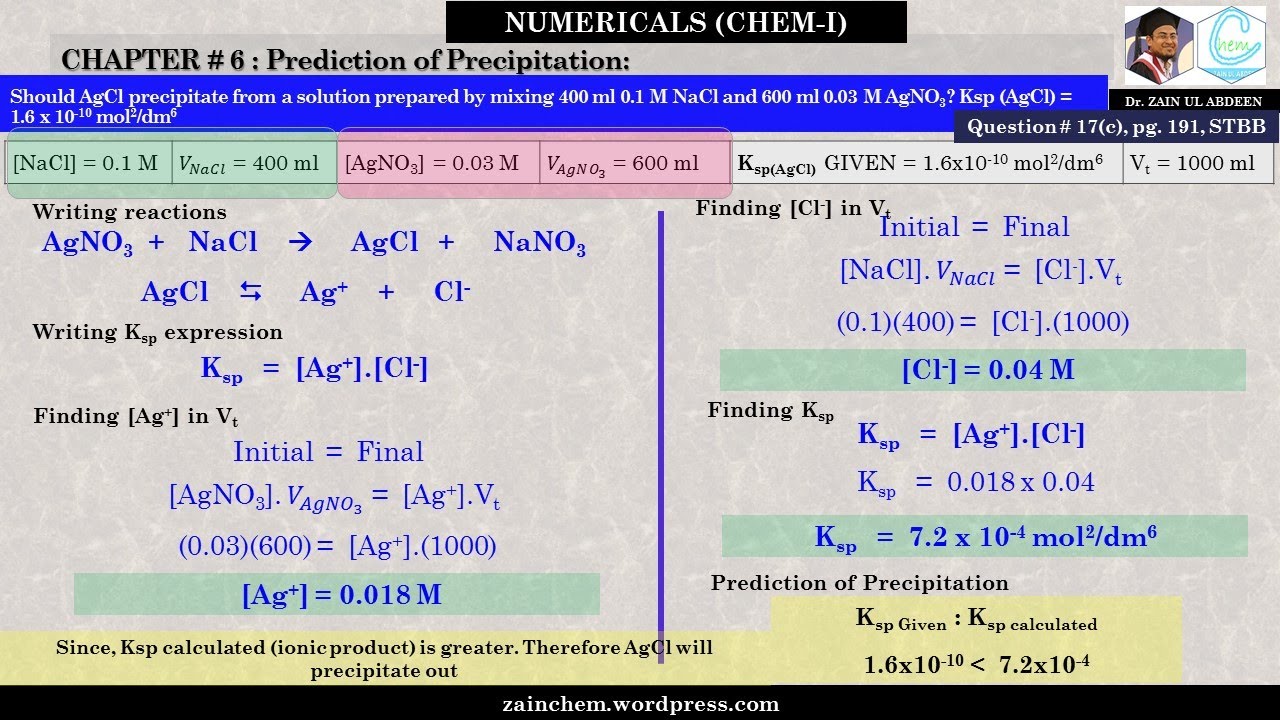
Previous work has focused on using direct neural network models for weather data, extending neural forecasts from 0 to 8 hours with the MetNet architecture, generating continuations of radar data for up to 90 minutes ahead, and interpreting the weather information learned by these neural networks. Within weather forecasting, deep learning techniques have shown particular promise for nowcasting - i.e., predicting weather up to 2-6 hours ahead. As the computation progresses, the network processes an ever larger context from the input and makes a probabilistic forecast of the likely future weather conditions. Illustration of the computation through MetNet-2. These approaches also have the potential to increase the frequency, scope, and accuracy of the predicted forecasts. Rather than incorporating explicit physical laws, deep learning models learn to predict weather patterns directly from observed data and are able to compute predictions faster than physics-based techniques. Such methods are constrained by high computational requirements and are sensitive to approximations of the physical laws on which they are based.ĭeep learning offers a new approach to computing forecasts. Weather forecasts typically rely on traditional physics-based techniques powered by the world’s largest supercomputers. The application of deep learning models to weather forecasts can be relevant to people on a day-to-day basis, from helping people plan their day to managing food production, transportation systems, or the energy grid. It will serve as a module for a new nowcasting model in the framework of the SPP 1167 Project Bundle an Nowcasting.Posted by Nal Kalchbrenner and Lasse Espeholt, Google Researchĭeep learning has successfully been applied to a wide range of important challenges, such as cancer prevention and increasing accessibility. A linearized flow and precipitation model is adapted to the observed flow fields and precipitation fields over complex terrain. The extent to which orographic enhancement of precipitation over various mountain ranges in Europe can be expressed by these numbers is assessed. It is aimed to apply a reduced description of basic states of the flow by means of dimensionless numbers, such as Froude numbers, Rossby number or mountain aspect ratio, an the basis of measurement and simulation data.
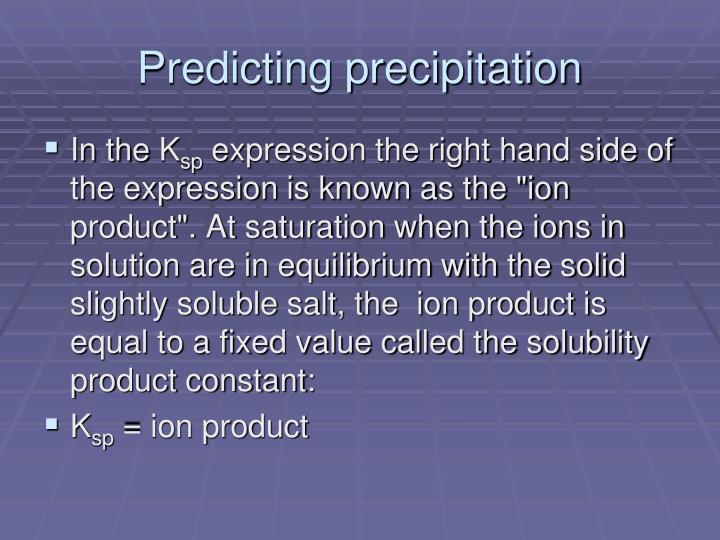
The reliability with which flow fields can be represented by numerical model simulations will be investigated by data comparison. For this reason, the analysis will first be devoted to identify the effect of flow dynamics an precipitation, such as flow over or around obstacles as well as flow blocking occurring over low mountain ranges during widespread precipitation events. Orographic influences and diabatic effects due to phase transformations modify the flow field in a way which is decisive for the orographically induced vertical wind field and, consequently, the intensity of orographically induced precipitation. Based an these results, a simplified module for nowcasting purposes of stratiform precipitation will be developed.
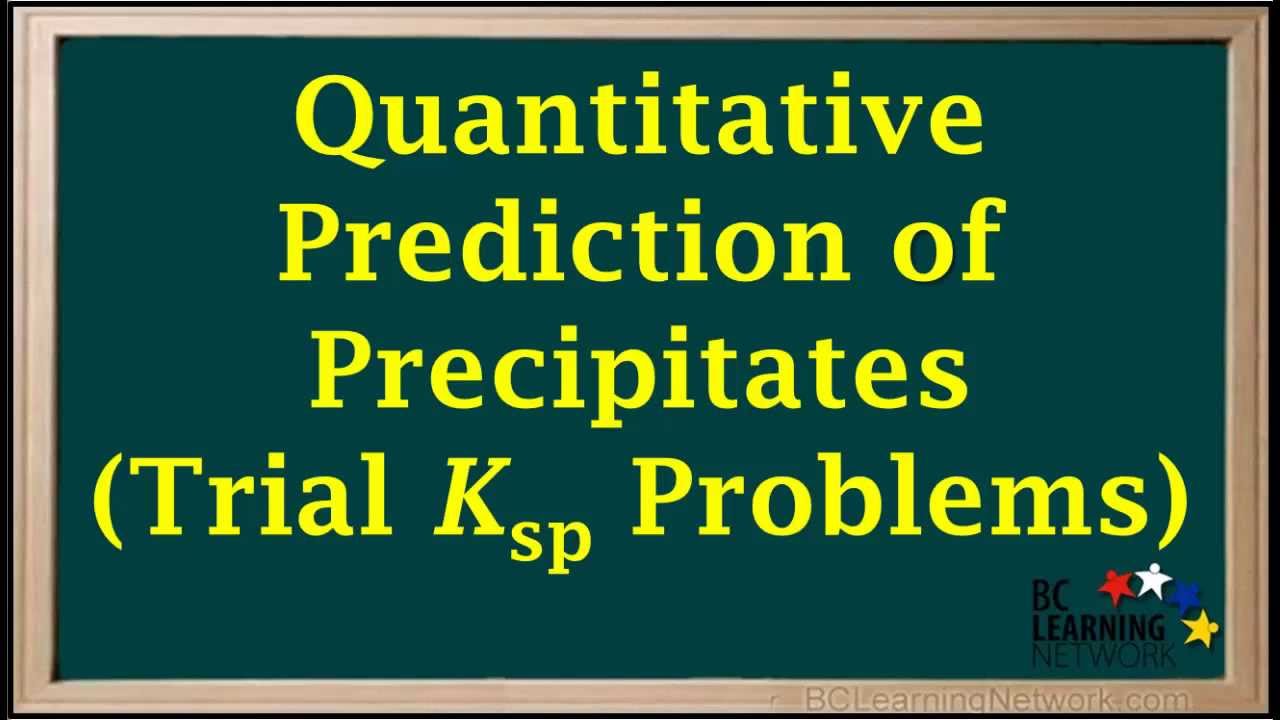
The connection between orographically influenced air flow and stratiform precipitation will be studied within the framework of the project an the basis of measured data and model simulations.
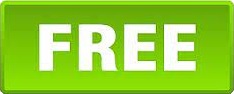